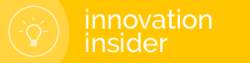
New algorithms to reduce relapse, readmission rates
08/13/21 11:50:amWith increasing relapse and readmission rates nationwide, Rogers is developing machine learning algorithms to predict which patients are likely to not respond to treatment. To overcome traditional outcome prediction limitations, Rogers is taking advantage of modern artificial intelligence methodology to assist with clinical decision and prediction.
Led by the Clinical Effectiveness team, the first completed algorithm – focusing on Depression Recovery – will be piloted in the fall. The goal is to ultimately develop algorithms for each service line and to implement them system-wide.
“The whole country is going through a mental health and addiction crisis,” says Jessica Cook, director, Clinical Effectiveness. “Patients are having more severe outcomes and less improvement. We want to leverage data and data science to help the clinical teams in being able to support their patients in recovery.”
“The idea is to study the massive volume of existing outcome data and understand the pattern of response so we can predict symptom severity,” Dr. Lee says. “This will be really useful for the clinical teams to create more personally effective treatment plans and maximize success for our patients.”
How the algorithms work
To develop the algorithms, a variety of analytics procedures were used to identify important clinical features relevant for outcome prediction. For the prediction of the primary outcome index, Rogers adopted one of the most advanced machine learning approaches called “deep neural network.”
Dr. Lee’s team used TensorFlow to develop these deep neural network models for predicting treatment outcome data. A framework developed by Google Brain team, TensorFlow has been increasingly utilized in various healthcare and genomics projects.
The algorithms then make predictions using data from baseline assessments collected at the time of admission. Each service line has its own primary outcome measures, such as the Quick Inventory of Depressive Symptomatology Self Report (QIDS) used by Depression Recovery.
According to Dr. Lee, the forecasted severity of the target symptom will allow Rogers to predict how well each patient will respond to treatment and what percentage of symptom reduction would be achieved based on the patient’s clinical profile at the time of admission.
The algorithms also offer detailed information on what clinical features would heavily contribute to the reduction of symptoms, which will assist our clinical care providers to optimize and individualize the treatment plan for each patient.
Leveraging the predictions to inform treatment plans
“Now more than ever, there is a need for our services,” says Rachel Leonard, PhD, executive director of Clinical Services, Regional Division, and the leader of the Depression Recovery clinical advisory board. “Seeing the expected trajectory for any given patient could be helpful in both planning duration of treatment and identifying patients who need additional supplemental intervention to enhance outcomes.”
Additionally, the algorithms could inform future protocol development and the impact of comorbidity. “Any tools we can use to enhance our decision-making and treatment we’re providing are very welcome,” Dr. Leonard says.
Only at Rogers
The algorithm development is unique to Rogers and one of the aspects that sets us apart from other behavioral healthcare organizations, according to Jessica.
“We believe we have the largest mental health database in the country – maybe even in the world,” Jessica says, referencing the one million patient self-assessments Rogers completes every year. “We are known for our measurement-based care that allows us to amass data that can predict patient outcomes.”
In fact, Rogers’ growing dataset will enable Dr. Lee and Dr. Lotfi – and their team including Jessica; Brian Kay, PhD, vice president, Continuous Improvement; and biostatistician Dalton Kick – to further refine the algorithms.
“As we collect more data and treat more patients, we will be able to make the models more accurate and sophisticated in the future by understanding the complex pattern of treatment outcome data better,” Dr. Lee says. “We don’t look at this as a one-time deal. This is just the beginning of our continuing and exciting endeavor to improve patient care through advanced data-analytic approaches.”